Understanding HDABL: A Comprehensive Guide To High-Dimensional Analysis Of Biological Data
**In the world of bioinformatics and systems biology, the term HDABL has emerged as a crucial concept for researchers looking to analyze complex biological data effectively.** This article aims to provide a detailed overview of HDABL, its applications, and its significance in modern biological research. By the end of this article, readers will have a thorough understanding of what HDABL entails and how it can be utilized in their studies.
The growing complexity of biological data, driven by advancements in technologies such as genomics, proteomics, and metabolomics, necessitates the development of robust analytical methods. HDABL, or High-Dimensional Analysis of Biological Data, is one such method that allows researchers to uncover hidden patterns and relationships within large datasets. In this guide, we will explore the foundational principles of HDABL, its methodologies, and its practical applications across various fields of biology.
As the field of biological research continues to evolve, the ability to analyze high-dimensional data effectively will be paramount. HDABL not only enhances our understanding of biological systems but also paves the way for new discoveries that could lead to significant advancements in medicine and biotechnology. This article will serve as a valuable resource for those interested in delving deeper into the intricacies of HDABL.
Table of Contents
- What is HDABL?
- Importance of HDABL in Biological Research
- Methodologies Used in HDABL
- Applications of HDABL
- Challenges and Limitations of HDABL
- Future of HDABL
- Case Studies in HDABL
- Conclusion
What is HDABL?
HDABL, or High-Dimensional Analysis of Biological Data, refers to a set of statistical techniques and computational methods designed to analyze complex biological datasets that involve numerous variables. These datasets can arise from various biological experiments, such as gene expression profiling, proteomics, and metabolomics.
High-dimensional data presents unique challenges due to the vast number of variables relative to the number of observations. Traditional statistical methods often fall short in such scenarios, leading to overfitting and inaccurate conclusions. HDABL aims to address these issues by employing advanced statistical techniques that can handle the complexity of the data.
Importance of HDABL in Biological Research
Understanding the importance of HDABL is crucial for researchers in the life sciences. Here are several key reasons why HDABL is essential:
- Enhanced Data Interpretation: HDABL allows for a better understanding of complex biological systems by identifying patterns and relationships within the data.
- Improved Predictive Models: By utilizing high-dimensional data effectively, researchers can develop more accurate predictive models for biological phenomena.
- Facilitation of Biomarker Discovery: HDABL can aid in the identification of potential biomarkers for diseases, leading to improved diagnostic and therapeutic strategies.
- Integration of Multi-Omics Data: HDABL provides a framework for integrating various types of biological data, such as genomics, transcriptomics, and proteomics.
Methodologies Used in HDABL
Several methodologies are commonly employed in HDABL to analyze high-dimensional biological data. These include:
1. Dimensionality Reduction Techniques
Dimensionality reduction techniques such as Principal Component Analysis (PCA) and t-Distributed Stochastic Neighbor Embedding (t-SNE) are widely used in HDABL to reduce the complexity of the data while preserving important information.
2. Machine Learning Algorithms
Machine learning algorithms, including supervised and unsupervised learning methods, play a crucial role in HDABL. These algorithms can help identify patterns and make predictions based on high-dimensional data.
3. Statistical Modeling
Statistical models such as generalized linear models (GLMs) and Bayesian approaches are often utilized to analyze high-dimensional datasets, allowing researchers to account for various confounding factors.
4. Network Analysis
Network analysis methods are employed to visualize and understand complex interactions among biological entities, such as genes and proteins, within high-dimensional datasets.
Applications of HDABL
HDABL has a wide range of applications in various fields of biological research, including:
- Genomics: HDABL techniques are used to analyze gene expression data, helping researchers identify genes associated with specific diseases.
- Proteomics: In proteomics, HDABL allows for the identification of protein interactions and pathways involved in biological processes.
- Metabolomics: HDABL is applied in metabolomics to analyze metabolic profiles and understand their role in health and disease.
- Clinical Research: HDABL methods facilitate the discovery of biomarkers for diseases, aiding in the development of personalized medicine.
Challenges and Limitations of HDABL
Despite its advantages, HDABL also faces several challenges and limitations:
- Overfitting: The high dimensionality of the data can lead to overfitting, where models perform well on training data but poorly on unseen data.
- Computational Complexity: Analyzing high-dimensional data can be computationally intensive, requiring advanced algorithms and significant processing power.
- Data Quality: The accuracy of HDABL results is highly dependent on the quality of the input data. Poor-quality data can lead to misleading conclusions.
Future of HDABL
The future of HDABL looks promising, with ongoing advancements in computational methods and data collection technologies. As biological datasets continue to grow in complexity, the development of more sophisticated HDABL techniques will be essential. Furthermore, the integration of artificial intelligence and machine learning into HDABL methodologies is expected to enhance data analysis capabilities significantly.
Case Studies in HDABL
Several notable case studies highlight the successful application of HDABL in biological research:
- Case Study 1: A study utilizing HDABL methods to identify gene expression profiles associated with breast cancer, leading to novel therapeutic targets.
- Case Study 2: Research employing HDABL to analyze proteomic data from Alzheimer's disease patients, uncovering potential biomarkers for early diagnosis.
Conclusion
In conclusion, HDABL represents a powerful framework for analyzing high-dimensional biological data. Its importance in modern biological research cannot be overstated, as it enables researchers to uncover hidden patterns and relationships that would otherwise remain obscured. As the field continues to evolve, embracing HDABL methodologies will be crucial for driving innovation and discovery in biology. We encourage readers to explore further into HDABL and consider its applications in their research.
We invite you to leave your thoughts in the comments below and share this article with fellow researchers interested in high-dimensional analysis. For more insights and articles on related topics, feel free to explore our website.
Betty Loh Ti: The Life And Legacy Of A Pioneering Actress
Unveiling The Life Of Mel Raido: A Journey Through His Career And Impact
Agatha Vega: A Comprehensive Biography Of The Rising Star

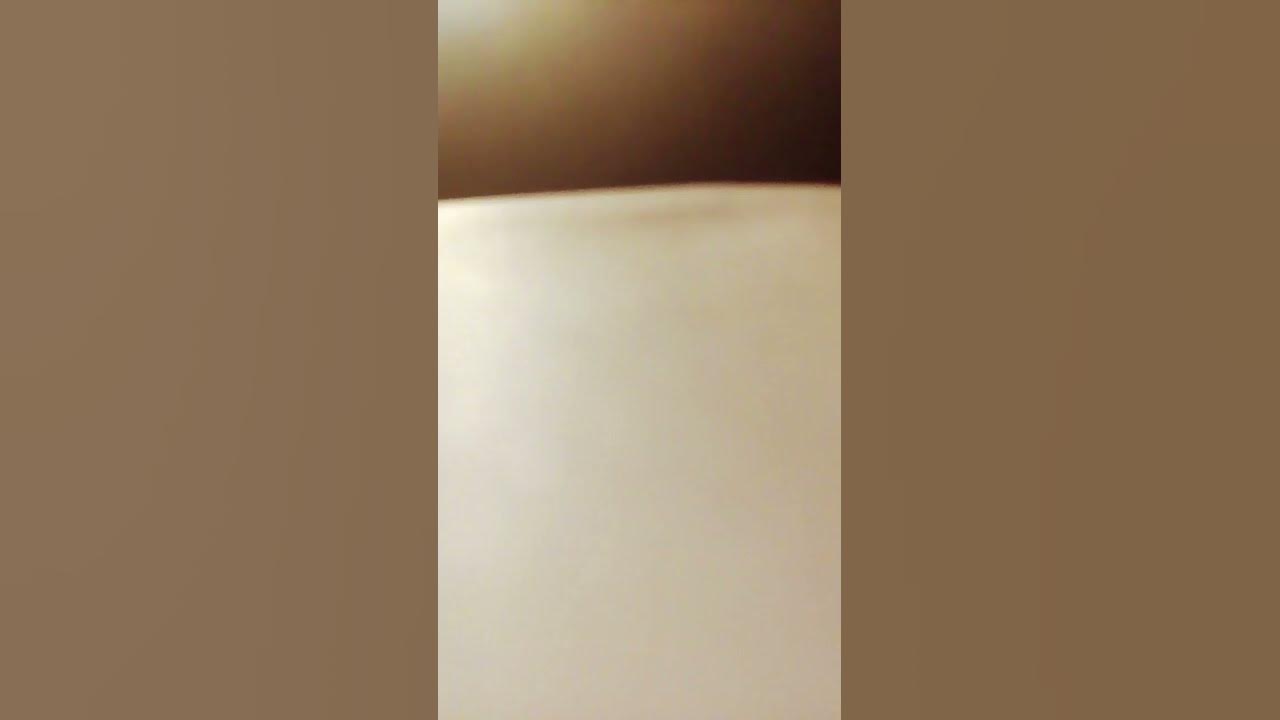
